2025/05/06(Tue) 10:30 -12:00 一樓演講廳 1F, Auditorium
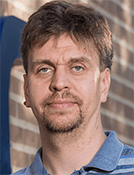
Title
Uncovering the mechanisms of pattern formation in myxobacteria with modeling and machine-learning
Speaker
Dr. Oleg Igoshin (Professor of Bioengineering, of Chemistry and of Biosciences, Rice University)Abstract
Collective cell movement is critical to the emergent properties of many multicellular systems, including microbial self-organization in biofilms, wound healing, and cancer metastasis. However, even the best-studied systems lack a complete picture of how diverse physical and chemical cues act upon individual cells to ensure coordinated multicellular behavior. Myxococcus xanthus is a model bacteria famous for its coordinated multicellular behavior resulting in dynamic pattern formation. For example, when starving, millions of cells coordinate their movement to organize into fruiting bodies – aggregates containing tens of thousands of bacteria. Relating these complex self-organization patterns to the behavior of individual cells is a complex, reverse-engineering problem that cannot be solved solely by experimental research. In collaboration with experimental colleagues, we use a combination of image processing, agent-based modeling, soft-matter physics, and kinetic theory to uncover the mechanisms of emergent collective behaviors.
In more recent developments, to quantify and compare self-organization dynamics systematically, we also employ the power of AI. We trained a deep-learning model to find a low-dimensional representation of brightfield microscopic images. Using a ResNet as an encoder, we compressed each image crop into a 13-dimensional feature vector. To validate this compression, we employed a StyleGAN2 as a generator to reconstruct high-resolution images from the feature vectors. During training, a Siamese network assessed the phenotypic similarity between input and reconstructed images. As a result, we obtained a model capable of reconstructing images that appear indistinguishable from input images in terms of phenotype. With the 13-dimensional features extracted, we developed a distance metric and 2-D graphical representation to compare 24-hour movies based on their self-organization dynamics. Our findings demonstrate the potential of deep learning methods in extracting emergent patterns in cellular systems, paving the way for future research in bacterial self-organization and system biology.
Poster
Language
演講語言 (Language): in English
Hosted by