2020/06/09(Tue) 15:00 -17:00 一樓演講廳 1F, Auditorium
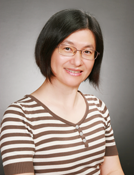
Title
Applications and Extensions of Principal Component Analysis: from a Top to Protein Structure Determination
Abstract
Most physicists first meet principal component analysis (PCA) in the context of ‘moment of inertia’ where PCA is employed to search the principal axis of a spinning top. In data science, PCA is arguably the most widely used dimension-reduction method for vector-type data. When applied to a sample of images, PCA requires vectorization of the image data, which in turn entails solving an eigenvalue problem for the sample covariance matrix. We propose herein a two-stage dimension reduction (2SDR) method for image reconstruction from high-dimensional noisy image data. The first stage treats the image as a matrix, which is a tensor of order 2, and uses multilinear principal component analysis (MPCA) for matrix rank reduction and image denoising. The second stage vectorizes the reduced-rank matrix and achieves further dimension and noise reduction. Simulation studies demonstrate excellent performance of 2SDR. Applications to cryo-EM (cryogenic electronic microscopy), which has revolutionized structural biology, organic and medical chemistry, cellular and molecular physiology in the past decade, are also provided and illustrated with benchmark cryo-EM datasets.
Poster
Download:
Language
演講語言 (Language): in Mandarin
Hosted by